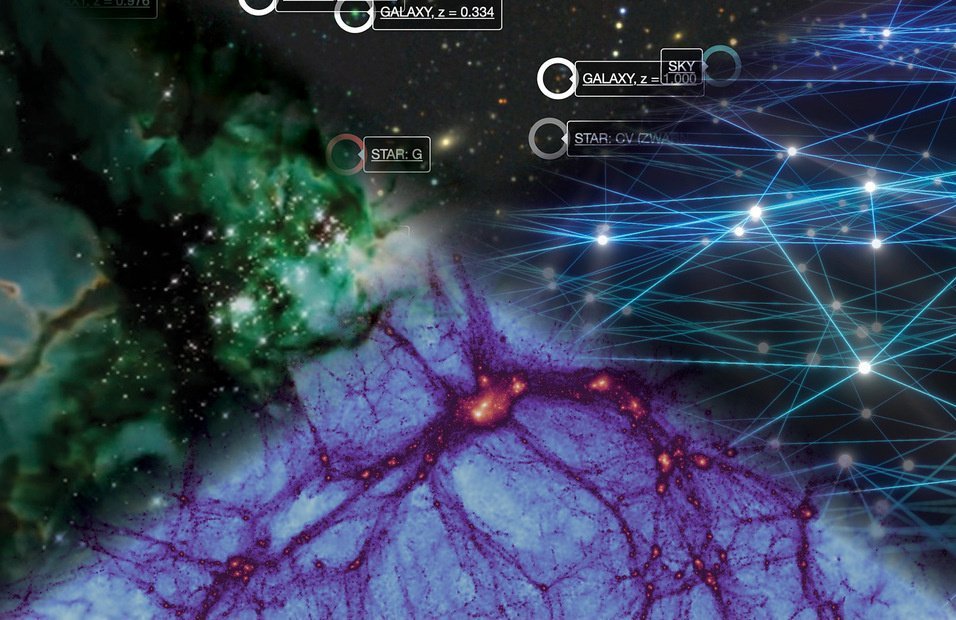
Upcoming events

May 5-9: AI Bootcamp For Astronomers
The boot camp will be held May 5-9 at the University of Texas at Austin. The boot camp aims to provide a broad overview of AI methods and use hands-on exercises to prepare students to apply AI methods to scientific applications in a high performance computing environment. This boot camp has a special focus on challenges in the domain of astronomy/astrophysics.
Please note that attending this boot camp assumes you have a basic proficiency in Python and have an interest in astronomy data and/or astronomy applications.
Participants are expected to attend all five days of the boot camp.
Bootcamp participants will join the conference at 4pm Tuesday-Thursday for highlighted talks.
Registration
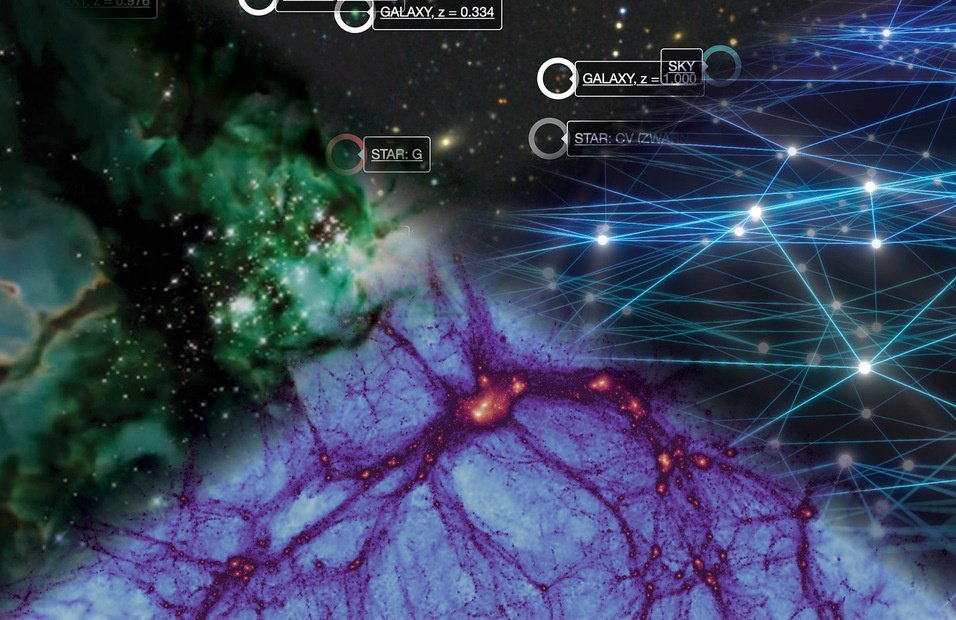

Hybrid Seminar - Exploring the LLM universe for astronomy research
CosmicAI Hybrid Seminar Series
Title: Exploring the LLM universe for astronomy research
Speaker: Dr. Jessy Li and Sebastian Joseph
Date: Wednesday, April 23rd 2025
Time: 1:00 PM CST
Venue: PMA 15.216B at UT Austin
Zoom: https://utexas.zoom.us/j/95289318277?pwd=G8WIQ9LenT54Qx4xB3OV1jiYh21Ja8.1
Meeting ID: 952 8931 8277
Passcode: 953649
Abstract: From ChatGPT to Deep Research, the pace of foundation models of AI has generated much excitement as well as reckoning: what are LLMs capable of? What are their limitations? How far can we push for AI models for research? One of the core missions of the Explorable Universe group within CosmicAI is to explore these questions for code LLMs.
In this talk, we will first present a primer that explains how LLMs are trained, especially within the context of our own code LLM training experience, thereby scoping the capabilities of these models. Next, we discuss our recent efforts to build a new coding benchmark that tests the limitations of LLMs, focusing on code execution and visualizations.
Speaker Bios
Jessy Li is an associate professor of Natural Language Processing and Computational Linguistics in the Department of Linguistics at UT Austin. She is the AI lead of the Explorable Universe group in CosmicAI.
Sebastian Joseph is a first-year Ph.D. student in Computer Science at UT Austin, advised by Prof. Jessy Li. His research focuses on making large language models more factual, understandable, and adaptable for expert domains.

Hybrid Seminar -Transcending the Limits of Astrostatistics with Machine Learning Methods
CosmicAI Hybrid Seminar Series
Title: Transcending the Limits of Astrostatistics with Machine Learning Methods
Speaker: Yuan-Sen Ting, Associate Professor at Ohio State | Adjunct Scientist at Max Planck Institute
Date: Wednesday, April 9 2025
Time: 1:00 PM CST
Venue: PMA 15.216B at UT Austin
Abstract:
Recent advancements in astronomical instrumentation have led to an unprecedented influx of data, revolutionizing the field of astronomy. However, the inherent complexity and multi-dimensionality of astronomical observations, ranging from intricate imaging of weak lensing, reionization, and protoplanetary disks to the comprehensive analysis of galaxy mergers across cosmic history, pose significant challenges to traditional astrostatistical methods.
In this colloquium, I will discuss two distinct machine learning approaches aimed at tackling these complex astronomical systems. First, I will explore the Mathematics of Information, focusing on how machine learning can optimize information compression and extract higher-order moments in stochastic processes.
Second, I will introduce a Generative AI paradigm, demonstrating how generative models, such as normalizing flows and diffusion models, enable precise modeling of astronomical datasets, facilitating accurate inferences on intricate astronomical systems. By leveraging these cutting-edge machine learning techniques, we can transcend the limitations of conventional astrostatistics, furthering making robust inferences on complex astronomical systems.
Speaker Bio
Yuan-Sen is an Associate Professor of Astronomy at Ohio State University and an Adjunct Scientist at the Max Planck Institute for Astronomy. He earned his Ph.D. in Astrophysics and Astronomy from Harvard and has held prestigious fellowships, including the NASA Hubble and Carnegie-Princeton Fellowships. His research leverages deep learning and AI for astrophysical data modeling, resulting in over 200 publications and a Nature cover story. Passionate about science outreach, he has organized major astronomy conferences in Malaysia, written for leading newspapers, and created popular TED-Ed videos.
Recording link here

Hybrid Seminar - Structure-preserving, Low-Parameter, Interpretable, Operator Learning for Surrogate Modeling
CosmicAI Hybrid Seminar Series
Title: Structure-preserving, Low-Parameter, Interpretable, Operator Learning for Surrogate Modeling
Speaker: Varun Shankar, Assistant Professor in the Kahlert School of Computing at the University of Utah
Date: March 26th, 2025
Time: 12pm MST (1 pm CST)
Venue: WEB 3780 | Evans Conference Room
Abstract: Scientific machine learning (SciML) is a relatively new scientific discipline that weds scientific computing and high-performance computing with carefully designed machine learning (ML) techniques. In the context of astrophysics, SciML has been applied to galaxy classification and identification, outlier detection, and uncertainty quantification.
Within SciML, operator learning is a rapidly emerging and powerful new paradigm for surrogate modeling across engineering and the sciences, with recent successes in climate modeling, material design, and carbon sequestration problems (to name a few). In this talk, I will present a unified framework that encompasses many operator learning paradigms and use this to present three advancements in operator learning: (1) the Kernel Neural Operator (KNO), a generalization of the Fourier neural operator that allows for greater flexibility in kernel choices and local spatial adaptivity while inherently using far fewer trainable parameters; (2) the ensemble DeepONet, a generalization to Deep Operator Networks that enables the incorporation of spatial adaptivity directly into a set of basis functions; and (3) a new operator learning paradigm based on kernel approximation that analytically preserves the divergence-free property and requires minimal training, all while achieving state-of-the-art performance on incompressible flow problems.
Based on these recent advances, I will argue that operator learning has the potential to positively impact astrophysics through trustworthy, rapid, and interpretable surrogate models for multiscale simulations of magnetohydrodynamics (MHD) and numerical general relativity (GR) and for inverse problems such as physical parameter estimation.
Watch the recording

Hybrid Seminar - Science with massive radio datasets enabled using AI/ML
CosmicAI Hybrid Seminar Series #4
Presenter: Dr. Mark Lacy, Scientist, National ALMA Science Center, at NRAO Charlottesville
Date: Wednesday, March 12, 2025
Time: 1:00 pm CST
Venue: NRAO ER-Auditorium
Link to seminar recording
Abstract: In common with other fields of astronomy, radio astronomy involves managing and analyzing large quantities of data. New and upcoming facilities such as the ALMA WSU and ngVLA will increase these data volumes and their complexity by 1-2 orders of magnitude. AI/ML methods can help in several ways during the process of going from an observing proposal to science. Language models can help sort thousands of proposals received for telescope time for review and setting up observations and processing pipelines. Numerically-based techniques can be used to assist with processing and analyzing the ~1-100TB datasets produced by current and future facilities.Last year (and restarting this year) we have been holding meetings at NRAO to discuss ways in which AI/ML can help with aspects of Observatory functions. This has been given new impetus by the award of the Cosmic AI Institute to a consortium including NRAO. In this talk I will summarize the outcomes of these meetings and present some of the ideas that arose from our discussions and some of the challenges that arise from using these techniques in an Observatory setting. I will also show some examples of AI/ML applied to radio astronomy data for both quality assurance and scientific purposes by ourselves and other groups working on data from the VLA Sky Survey.

Hybrid Seminar - Cosmological Emulators for High-Dimensional Inference
Presenter: Dr. Mahdi Qezlou, HETDEX-Cosmology Postdoctoral Fellow, University of Texas at Austin
Date: Wednesday, February 26, 2025
Time: 1:00 pm CST
Venue: POB 4.304
Zoom: https://utexas.zoom.us/j/96542873118
Meeting ID: 965 4287 3118
Passcode: 750886
One tap mobile +13462487799,,96542873118# US (Houston) +16694449171,,96542873118# US
Abstract:
Continuing the recent theme at the CosmicAI talks, I discuss “surrogate modeling” for inferring cosmological and astrophysical parameters with the help of machine learning. The available and upcoming observations require accurate simulations of the complex physical processes, including the non-linear gravity and gas dynamics. Using these detailed simulations at each Monte Carlo Markov Chain (MCMC) step in an inferential framework is computationally prohibitive. To overcome this, machine learning (surrogate) models can be trained to learn the map from the parameters of interest to the observables.
In particular, I will highlight a multi-fidelity Gaussian Process framework designed to maximize the constraining power on a high-dimensional input parameter space while minimizing the computational cost. I will also demonstrate how these emulators enable unprecedented accuracy in parameter inference, paving the way for robust scientific discoveries with current and future cosmological surveys.

Hybrid Seminar - Some advances in simulation-based inference: Calibration, aggregation, and model check
CosmicAI Hybrid Seminar Series
Title: Some advances in simulation-based inference: Calibration, aggregation, and model check
Presenter: Dr. Yuling Yao, Assistant Professor in the Department of Statistics and Data Sciences at the University of Texas at Austin. yulingyao.com.
Venue: POB 4.304 (UT Austin)
Join Zoom Meeting: utexas.zoom.us/j/95502700680 (Meeting ID: 955 0270 0680, Passcode: 745761)
Abstract:
Simulation is a powerful way to specify models in modern scientific computing, while the likelihood-free setting imposes challenges for inference and calibration. To start, I present a cosmology example of galaxy clustering analysis using simulation-based inference and normalizing flows. I present three recent advances in simulation-based inference:
(1) “discriminative calibration” develops a general classifier approach to check Bayesian computation including simulation-based inference and Markov chain Monte Carlo. The classifier performance is a consistent estimate of a family of divergence measures, including the classical classifier two-sample test as a special case.
(2) To incorporate posterior approximations from different inference algorithms or flow architectures and improve the final inference quality, I present “simulation-based stacking”, a general framework to combine probabilistic inferences.
(3) Yet even when the inference is perfect, the simulation model is often an approximation to the nature. I present “simulation-based posterior predictive check”, a framework to check if the simulation model does a good job of capturing relevant aspects of the data, such as means, standard deviations, and quantiles. This new predictive check p-value is ensured to be frequentist-calibrated under the null, making it particularly suitable for rigorously testing scientific hypothesis.
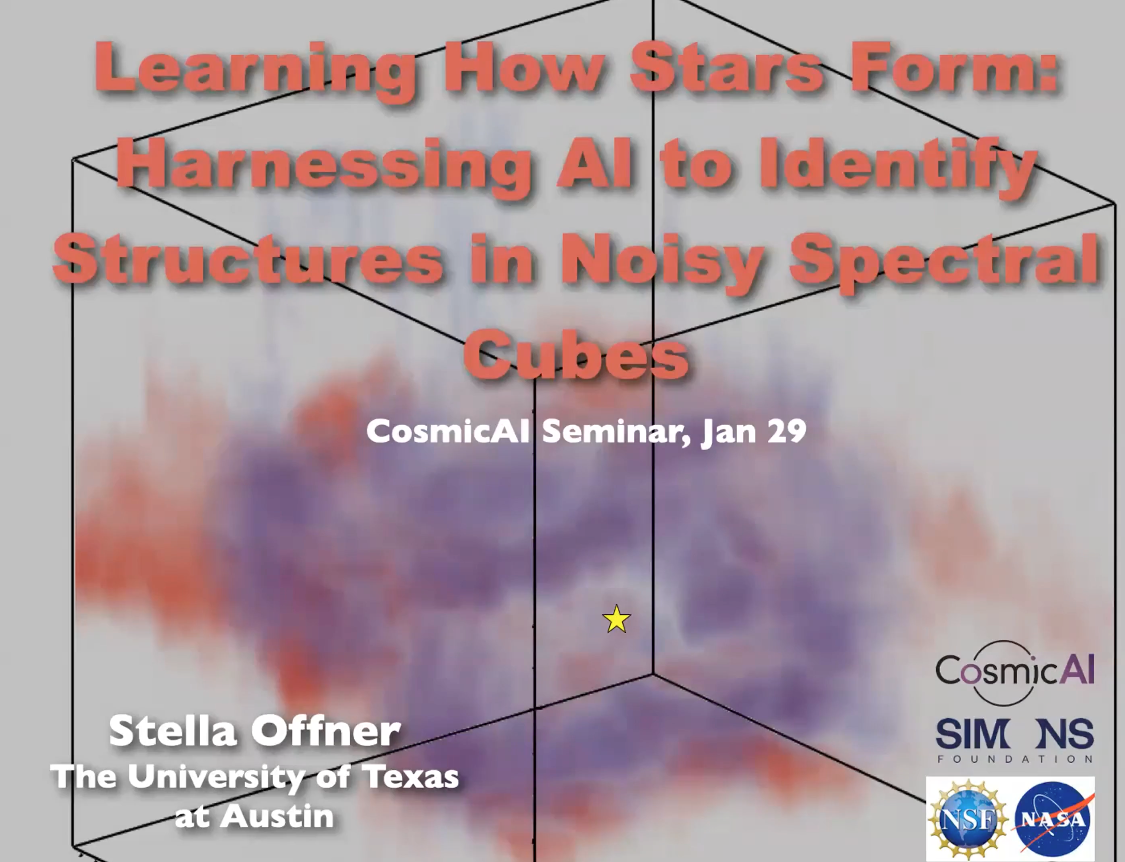
Hybrid Seminar: Learning how Stars Form: Harnessing AI to Identify Structures in Noisy Spectral Cubes.
Title: Learning how Stars Form: Harnessing AI to Identify Structures in Noisy Spectral Cubes.
Dr. Stella Offner and Dr. Josh Taylor
Abstract:
Star formation is messy! The process spans many orders of magnitude in scale and involves a variety of physical processes: gravity, magnetic fields, radiation, and turbulence. Young stars announce their presence by emitting radiation and ejecting high-velocity material, “stellar feedback,” which in turn shapes the surrounding natal environment.
Part 1: Stella will present the results from a 3-D convolutional neural network model, trained on full-physics numerical simulations, which can accurately identify stellar feedback features in molecular line spectral cubes. She will discuss the pros and cons of supervised approaches based on numerical simulations for data segmentation.
Part 2: Josh will present a new approach to identifying cohesive spectral structures in molecular line cubes. Multiview Prototype Embedding and Clustering (MPEC) is an integrated approach to simultaneously:
Learn prototypes of spectral data for efficient sample size reduction,
Embed these prototypes in a lower dimensional latent space generated by a Self-Organizing variant of UMAP, and
Cluster a graph representation of (learned) prototype similarity in both feature and latent spaces.
All facets of MPEC are self-parameterized, making it feasible for pipeline processing of spectral cubes. Josh will also discuss noisy cluster identification for spectral data using standard tools from statistical time series analysis.
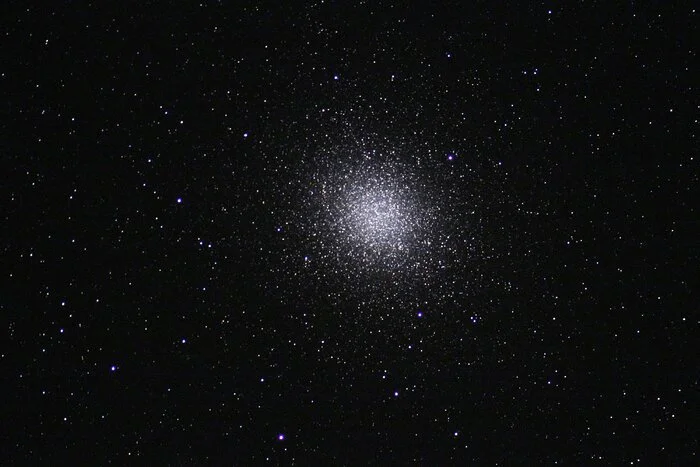
Wednesdays at 1pm CST: NSF-Simons CosmicAI Hybrid Seminar Series
This spring NSF-Simons AI Institute for Cosmic Origins (CosmicAI) is launching a new hybrid online/in-person seminar series for the community working at the intersection of AI and astronomy.
This seminar is intended to showcase research that is of broad interest and importance to AI developments in astrophysical research. This spring the primary in-person location will be at UT Austin, with some rotating guest seminars from researchers at other institutions.
Stella Offner, Director of NSF-Simons CosmicAI, and Josh Taylor will kick off the series on January 29: “Learning how Stars Form: Harnessing AI to Identify Structures in Noisy Spectral Cubes.”
Image Credit: KPNO/NOIRLab/NSF/AURA/Blythe Guvenen
Galactic Quest: An AI Mission to Decode the Cosmos
Discover how recent and near-future AI developments will be able to accelerate discoveries related to the Universe and address the most basic human question: Where do we come from? Go deep into the possibilities of the cosmos with expert astronomers, data scientists, and more.
Speakers: Arya Farahi, Josh Taylor, Matt Lease, and Stella Offner.
More details and RSVP here including transit and parking information.
Events
Check out our calendar for details on hybrid seminars, the Cosmic Horizons Conference, Astro-AI Bootcamp, and more.